What You Need to Know About Disease Interception
- Titus Kaletta
- Jul 13, 2022
- 7 min read
Updated: Jun 19, 2023

Detecting, treating and even curing diseases before the patient develops any symptoms almost sounds too good to be true, but it is precisely what so-called ‘disease interception’ promises. Disease interception is an emerging field of research with implications for Alzheimer’s disease, cancer and certain other disorders. We discuss this concept and the use of artificial intelligence in this context.
One of the major challenges in modern healthcare is the early diagnosis and prevention of diseases. These challenges are amplified by an aging population and extended life expectations. Chronic progressive diseases such as Alzheimer's or cancer are difficult to cure, once the first clinical symptoms appear. Early detection of Alzheimer's or certain types of cancer before symptoms appear and thus actually prevent them would be the holy grail in healthcare: In fact, this could become possible in the future with the help of data analysis and artificial intelligence. The approach is called "disease interception". It would enable doctors and hospitals to diagnose patients as early as possible to detect and prevent their diseases and as such allow patients to seek medical care before developing the actual disease. What is the vision behind this new medical concept? Is it really new? We introduce this concept and discuss the challenges ahead.
Content
Disease Interception - a Specific Form of Secondary Prevention
Disease interception represents a new strategy for the prevention of diseases. In the case of Alzheimer's, interception means diagnosing it early before it causes any significant damage to the brain. But what is disease interception exactly? These four criteria set it apart from other prevention strategies such as cancer screening or genetic testing (1):
The disease has already begun but is still clinically unapparent,
The disease will almost certainly develop its full clinical picture without intervention,
There is only a certain window of opportunity to intercept and
An intervention is available and individualized.
For example, prostate carcinoma screening using the PSA test may help to detect a risk for the patient early, but an elevated PSA level does not mean, that a malign tumor will develop. The patient has this information of having a risk to develop prostate cancer but other then regular monitoring no interception is available today (preventive surgery would be considered as over-treatment). This type of prevention differs from disease interception, because it does not meet the second and fourth criterion (Nevertheless, the PSA test is a valuable diagnosis tool!). We want to elaborate on disease interception using Alzheimer's disease (AD) as case.
The Role of Disease Interception in Alzheimer’s Research
Alzheimer's disease (AD) is a good example, to show the usefulness of disease interception. Most AD patients develop the disease over the age of 65. Early diagnosis is carried out by mental tests and increasingly with bio-molecular marker assays for tau and beta-amyloid. The problem is, Alzheimer's disease begins long before any symptoms become apparent. AD patients will not notice symptoms during the early stages, nor will relatives and friends. But once AD is diagnosed, damage to the brain already occurred because the onset of the disease took place many years earlier.
A recent drug against AD again failed, despite particularly patients with early-stage AD being targeted, because damage to the brain was already present and the disease progression could not be halted. There is apparently a time window between a healthy brain and a diseased brain with clinical symptoms, which would offer the possibility to intercept. Unfortunately, neither the time window is known (it may very vary in duration between a few years and over a decade as well as it may begin at the age of 50 but also in later ages) nor the precise trigger for AD is known.
Intercepting Alzheimer’s means identifying people who may be at high risk for developing the disease, monitoring them more carefully with regular checkups and intercept (treat) before it can unfold. The idea is that catching the disease very early may allow for more effective treatments, milder interventions or even cure.
Artificial Intelligence to Leverage Disease Interception
While researchers have been looking for biomarkers for many years, employing artificial intelligence may be a new way to make this vision true. Recent advances in machine learning provide new tools to help with disease interception research. For example, one team of researchers led by Dr. Anke Diehl, Chief Transformation Officer of the University Medicine Essen, uses AI to predict the progression of Alzheimer’s disease in a group of patients based on their genetic data (2). "To find early indications of a disease, large amounts of data, Big Data, have to be collected and analyzed. Humans alone can not do this; artificial intelligence is needed to identify correlations that we may not even be aware of today". Obviously, the more data available over a longer period of time, the better and more accurate the results. The collection of these large volumes of data is currently still one of the sticking points, but so is standardization. Only when data is available in a standardized format, can it be evaluated using AI and machine learning (ML). So essentially, not just big data but rather smart data are needed. Typical data that could be used to train ML algorithms would be:
Structural data: Data not modified by humans, such as radiographic images.
Behavioral data: Standardized data that is influenced by human behavior
Genetic data: DNA or RNA sequences extracted from patient blood samples
Clinical chemistry data: blood testing and the use of more sophisticated but broader approaches e.g., metabolomics analysis
But it is not so straightforward. 1. Radiographic data derived by PET-Scanning of AD patients or patients with mild cognitive impairments are valuable to diagnose AD but this would be too late. Whether the complexity of this method would allow for broad screening of elderly populations is at question. 2. Behavioral data using the standard mental tests would be possibly too subtle and therefore too difficult for proper standardization. 3. Genetic data unless patients have familial AD with known mutations, we are back to genetic predisposition which may or may not lead to the disease. 4. The aforementioned biomarkers tau and beta-amyloid are not reliable enough to diagnose AD in pre-clinical stages. Furthermore, those, who are knowledgeable in AD research know, that there is a wealth of putative AD risk factors. And none of them alone would make a reliable biomarker or indicator to diagnose pre-clinical AD.
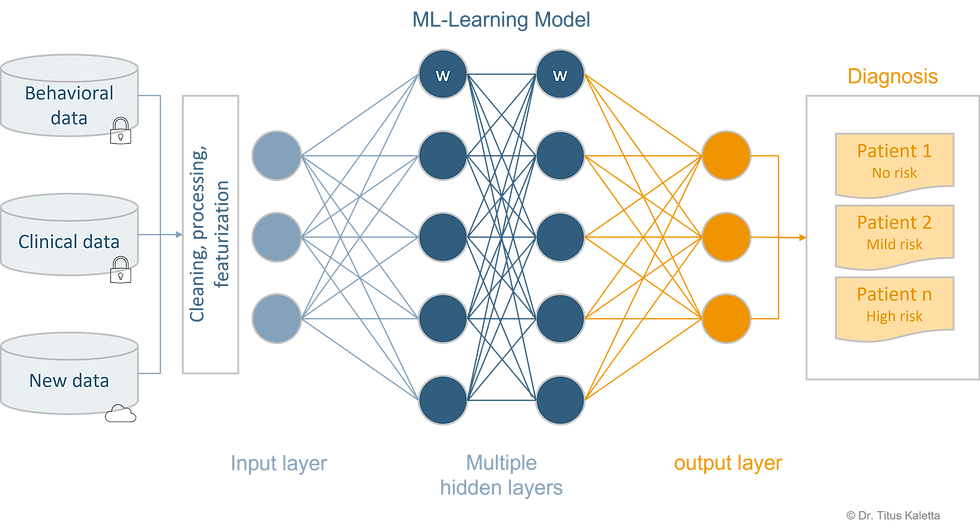
Figure 1. Simplified machine learning model: Collection of data -> processing of data > building the ML model -> deployment of model leading to diagnosis and risk assessment.
Here, AI could help to establish individual profiles for pre-clinical AD patients using a combination of indicators that alone would not be indicative. So, instead of one or a few markers with a defined cut-off to establish a diagnosis, AI would be able to generate various risk profiles with many also weak indicators for different patients.
A promising example is the use of speech biomarkers. The German start-up ki-elements employs AI to automatically analyze speech patterns, which are associated with early-onset AD. The data from this method can be highly standardized, but would be derived from individuals. The Israelian company Medial EarlySign takes this a step further: they develop different algorithms to detect subtle, early signs of disease in high-risk patients based on ordinary electronic health records and existing lab results.
Using AI for disease interception sounds brilliant particularly for very early and ambiguous signs. But we need to keep in mind, that also a treatment, an interception, is needed to cure the disease or at least delay its on-set.
Challenges for Disease Interception
Principally, the concept of disease interception can only work if
diagnosis of the disease before its onset with high certainty to avoid over-treatment
treatments regimes are available, that can intercept such a disease beyond the change of lifestyle, and
the readiness of a patient to cope with the diagnosis and accept a treatment regime.
A prominent example is Angelina Jolie. A mutation in a breast cancer gene, BCAR1, which is associated with a high risk to develop breast cancer was identified. She underwent precautionary a mastectomy. This was not an easy decision and she had a dilemma: either she accepts the mastectomy despite it is not for certain she would develop breast cancer or she rejects the surgery with the risk, the cancer would develop rapidly undetected and treatment would be very difficult. Disease interception might have been a solution to detect the onset of breast cancer early enough for immediate and effective treatment, but also to avoid any treatment if it would not develop.
Let's return to AD. For AD exists is no cure today. It is thus an ethical issue to forecast the occurrence of a disease but tell the patient there is nothing she or he could do. The concept of disease interception can only be successful, if it is possible to really intercept. Another point to consider is, that the side effects of treatment have to be very low or the duration of the treatment is so short that no significant side effects accumulate. For example, there is a debate about the right start of using statins to prevent coronary heart disease as not all patients tolerate statins well. Another challenge is to design appropriate clinical trials. Executing a clinical trial with clinically healthy patients using a new drug may raise ethical concerns as well.
Conclusion and Perspective
Disease interception is a promising approach, that could have a significant impact on treating and managing Alzheimer’s as well as other chronic-progressive diseases. However, it’s important to keep in mind that it is still an emerging field of research and there are several limitations to disease interception as a strategy. Using AI may help to find correlations that otherwise would be difficult to find by humans. Also, it could combine weak or indirect indicators to compute patient-specific risk profiles. The hope to find and intercept with the switch that starts the development of a disease might still be science fiction, but every month and year, a treatment regime begins earlier will improve the probability of stopping or delaying the disease.
Photo by shutterstock.
Comments